Although frequently interchanged in the self-driving vehicle space, it’s important to understand that automation and autonomous do not mean the same thing.
We can think of automation as a technology’s ability to perform rigid and repetitive tasks. The paths and functions of these technologies are usually programmed in advance and lack the ability to depart from that predetermined functionality — and when the unexpected happens, they usually require human intervention.
The Roomba is an example of this kind of automation. As the robotic vacuum meanders around your house, it knows that it needs to change directions when it hits a wall. What it might not know, however, is how to respond if it wanders outside.
On a larger scale, we can see automation with autopilot technologies in aviation. These systems have been programmatically designed to keep the plane level and headed in the right direction; however, any departure from that operation usually requires a pilot to take over.
By contrast, autonomous systems are designed to be far more self-directed. In most cases, these systems use artificial intelligence to develop an understanding of the rules of the domain it operates in. This higher level of intelligence enables the autonomous system to make its own judgment about how to react to various scenarios, enabling a fundamental capability that advanced autonomy creates: the ability to act under uncertainty. This ability is what allows autonomous systems to resolve conflicts where automated systems would quit.
For example, the technology underpinning Amazon Alexa’s voice recognition capabilities is called Natural Language Processing. Without artificial intelligence, developers would be required to pre-program every phoneme, word, and possible human sentence into an interactive voice agent, defining how Alexa should respond in advance.
Instead, thanks to Artificial Intelligence, Alexa can be taught to develop her own understanding of the relationship between human sounds and the response she should give. This makes her far more capable of responding naturally and in real-time to any human sentence that may be put in front of her.
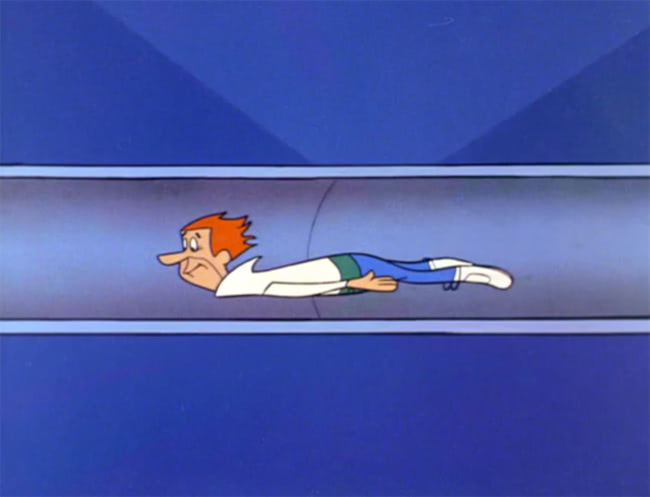
The Automated Train vs. the Autonomous Vehicle
In 1967, the first automated trains hit the tracks in London’s Underground Victoria Line. At first, operators were responsible for closing the doors and starting the trains — but once the command was issued, the train could pilot itself. Not only would it automatically stop at its next station, but it could also come to a stop if it detected an obstacle on the track.
Today, entire metros from Japan to Denmark run safely and efficiently without a human operator. In short, automated solutions beat autonomous solutions by 50 years.
Closed systems like those in a metro are perfect for automation — and it makes sense. To operate safely, trains must successfully complete just a handful of functions. They must go and stop, slow down around curves, and break when an obstacle crosses their path.
By contrast, cars must do many more things. Beyond the going and stopping, they have to make unprotected lefts, interpret pedestrian hand signals, recognize that a red blip a half a football field away is a stoplight, and counter-steer when they start to spin out.
They also have to do all this perfectly in all weather conditions at all times of the day.
As the list of these edge cases expands, programmatic automation simply can’t keep up. It’s not possible to define in advance all the possible scenarios that a car might face.
Instead, autonomous vehicle developers put artificial intelligence on the case, trusting the system itself to develop its own intuition about how to interpret and respond to the world in real-time.
In the case of warehouses, yards, or other ODDs (Operational Design Domains), deploying automated vehicles brings forth its own set of challenges due to the complexity of adapting these environments to work smoothly with automation. In many cases, operators have to completely rethink the operational flow, including the site layout, expensive infrastructure, how humans interact with different assets, etc.
By contrast, deploying autonomous vehicles removes the reliance of new deployments on expensive, highly customized, unscalable projects by leveraging the capabilities of more advanced vehicles. By bringing in a technology that can creatively and flexibly adapt to its environment, organizations can avoid site-wide overhauls and bring step-changes to their operational efficiency.
Cyngn’s DriveMod has been engineered with versatility in mind so that it can integrate with the long-tail of vehicle types and form factors. From grain swathers to stock chasers, our flexible architecture and universal autonomy solutions bring autonomous vehicle capabilities to a variety of vehicles, industries, and needs.
Whether retrofitting an existing fleet or integrating Cyngn into vehicles right off the factory floor, our customers achieve a return on their autonomous vehicle investment in about 8 months.